Beauty in Details: HSE University and AIRI Scientists Develop a Method for High-Quality Image Editing
Researchers from the HSE AI Research Centre, AIRI, and the University of Bremen have developed a new image editing method based on deep learning—StyleFeatureEditor. This tool allows for precise reproduction of even the smallest details in an image while preserving them during the editing process. With its help, users can easily change hair colour or facial expressions without sacrificing image quality. The results of this three-party collaboration were published at the highly-cited computer vision conference CVPR 2024.
Artificial intelligence is already able to generate and edit images using generative adversarial networks (GANs). The architecture consists of two independent networks: a generator that creates images and a discriminator that distinguishes between real and generated samples. These networks compete with each other, and a new stage in their development is the StyleGAN model. This model can generate images and modify specific parts based on user requests, but it has not been able to work with real photos or images before.
Researchers from the HSE AI Research Centre, the Artificial Intelligence Research Institute (AIRI), and the University of Bremen have proposed a method to quickly and efficiently edit real images. This StyleFeatureEditor approach consists of two modules: the first inverts (reconstructs) the original image, and the second edits this reconstruction. The results of these two steps are passed to StyleGAN, which generates the edited image based on the internal representations. The developers addressed some challenges that had been encountered in previous research. With a small set of representations, the network could edit the image well, but it lost some details from the original. However, with a larger set, all the details were preserved, but the network had difficulty transforming them correctly according to the task.
To solve this, the researchers proposed a new solution: the first module finds both large and small representations, while the second learns how to edit the larger ones using the smaller ones as reference.
However, to train these modules to accurately edit the representations, the neural network requires both real images and their edited versions.
‘We needed examples, such as the same face with different expressions, hairstyles, and details. Unfortunately, such image pairs do not exist at the moment. So, we came up with a trick: using a method that works with small representations, we created a reconstruction of a real image and an example of editing this reconstruction. Although the examples were relatively simple and without details, the model clearly understood how to make the edits,’ explains Denis Bobkov, one of the authors of the article, a research intern at the Centre of Deep Learning and Bayesian Methods of the AI and Digital Science Institute (part of the HSE Faculty of Computer Science), and a Junior Research Fellow at AIRI’s Fusion Brain Lab.
However, training only on generated (simple) examples leads to a loss of detail when working with real (complex) images. To prevent this, the researchers added real images to the training dataset, and the neural network learnt to reconstruct them in detail.
Thus, by showing the model how to edit both simple and complex images, the scientists created conditions under which the network could edit complex images more effectively. In particular, the developed approach handles adding new elements of style while preserving the details of the original image better than other existing methods.
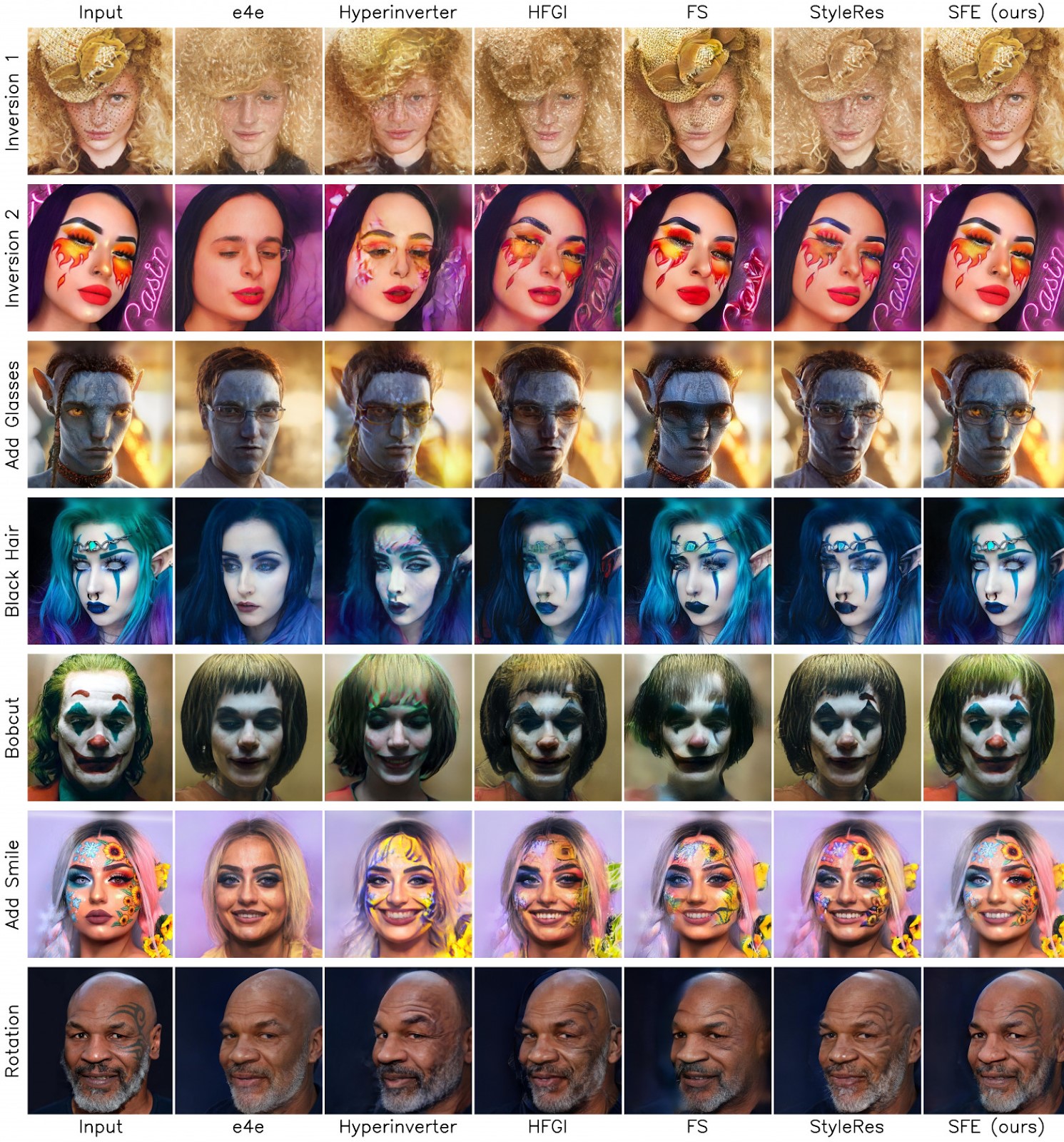
In the case of simple reconstruction (first row), StyleFeatureEditor accurately reproduced a hat, while most other methods almost completely lost it. The developed method showed the best results with additional accessories (third row): most methods could add glasses, but only the StyleFeatureEditor retained the original eye colour.
‘Thanks to this training technique on generated data, we have obtained a model with high editing quality and a fast processing speed due to the use of relatively lightweight neural networks. The StyleFeatureEditor framework requires only 0.07 seconds to edit a single image,’ says Aibek Alanov, Head of the Centre of Deep Learning and Bayesian Methods of the AI and Digital Science Institute (part of the HSE Faculty of Computer Science), and leader of the research group ‘Controlled Generative AI’ at AIRI's Fusion Brain Lab.
The research was funded by a grant from the Analytical Centre under the Government of the Russian Federation for AI research centres.
The research results will be presented at the Fall into ML 2024 conference on artificial intelligence and machine learning, which will take place at HSE University on October 25–26, 2024. Leading AI scientists will discuss the best papers published at top-tier (A*) flagship AI conferences in 2024. A demo of the developed method can be tried out on HuggingFace, and the source code is available on GitHub.
See also:
Scientists Examine Neurobiology of Pragmatic Reasoning
An international team including scientists from HSE University has investigated the brain's ability to comprehend hidden meanings in spoken messages. Using fMRI, the researchers found that unambiguous meanings activate brain regions involved in decision-making, whereas processing complex and ambiguous utterances engages regions responsible for analysing context and the speaker's intentions. The more complex the task, the greater the interaction between these regions, enabling the brain to decipher the meaning. The study has been published in NeuroImage.
Scientists Present New Solution to Imbalanced Learning Problem
Specialists at the HSE Faculty of Computer Science and Sber AI Lab have developed a geometric oversampling technique known as Simplicial SMOTE. Tests on various datasets have shown that it significantly improves classification performance. This technique is particularly valuable in scenarios where rare cases are crucial, such as fraud detection or the diagnosis of rare diseases. The study's results are available on ArXiv.org, an open-access archive, and will be presented at the International Conference on Knowledge Discovery and Data Mining (KDD) in summer 2025 in Toronto, Canada.
Hi-Tech Grief: HSE Researchers Explore the Pros and Cons of Digital Commemoration
Researchers at HSE University in Nizhny Novgorod have explored how technological advancements are transforming the ways in which people preserve the memory of the deceased and significant events. Digital technologies enable the creation of virtual memorials, the preservation of personal stories and belongings of the deceased, interaction with their digital footprint, and even the development of interactive avatars based on their online activity. However, these technologies not only evoke nostalgia and provide a sense of relief but can also heighten anxiety and fear, and delay the process of accepting loss. The study has been published in Chelovek (The Human Being).
Scientists Find Out Why Aphasia Patients Lose the Ability to Talk about the Past and Future
An international team of researchers, including scientists from the HSE Centre for Language and Brain, has identified the causes of impairments in expressing grammatical tense in people with aphasia. They discovered that individuals with speech disorders struggle with both forming the concept of time and selecting the correct verb tense. However, which of these processes proves more challenging depends on the speaker's language. The findings have been published in the journal Aphasiology.
Implementation of Principles of Sustainable Development Attracts More Investments
Economists from HSE and RUDN University have analysed issues related to corporate digital transformation processes. The introduction of digital solutions into corporate operations reduces the number of patents in the field of green technologies by 4% and creates additional financial difficulties. However, if a company focuses on sustainable development and increases its rating in environmental, social, and governance performance (ESG), the negative effects decrease. Moreover, when the ESG rating is high, digitalisation can even increase the number of patents by 2%. The article was published in Sustainability.
Russian Scientists Develop New Compound for Treating Aggressive Tumours
A team of Russian researchers has synthesised a novel compound for boron neutron capture therapy (BNCT), a treatment for advanced cancer that uses the boron-10 isotope. The compound exhibits low toxicity, excellent water solubility, and eliminates the need for administering large volumes. Most importantly, the active substance reaches the tumour with minimal impact on healthy tissues. The study was published in the International Journal of Molecular Sciences shortly before World Cancer Day, observed annually on February 4.
Scientists Discover Link Between Brain's Structural Features and Autistic Traits in Children
Scientists have discovered significant structural differences in the brain's pathways, tracts, and thalamus between children with autism and their neurotypical peers, despite finding no functional differences. The most significant alterations were found in the pathways connecting the thalamus—the brain's sensory information processing centre—to the temporal lobe. Moreover, the severity of these alterations positively correlated with the intensity of the child's autistic traits. The study findings have been published in Behavioural Brain Research.
Earnings Inequality Declining in Russia
Earnings inequality in Russia has nearly halved over the past 25 years. The primary factors driving this trend are rising minimum wages, regional economic convergence, and shifts in the returns on education. Since 2019, a new phase of this process has been observed, with inequality continuing to decline but driven by entirely different mechanisms. These are the findings made by Anna Lukyanova, Assistant Professor at the HSE Faculty of Economic Sciences, in her new study. The results have been published in the Journal of the New Economic Association.
‘When You Have a Lot to Do, You Find Time for Everything’
Egor Churaev specialises in neural networks. In an interview for the HSE Young Scientists project, he talked about his program for determining the emotions and engagement of online conference participants, his trip to Brazil, and his sports hobbies.
Russian Physicists Discover Method to Increase Number of Atoms in Quantum Sensors
Physicists from the Institute of Spectroscopy of the Russian Academy of Sciences and HSE University have successfully trapped rubidium-87 atoms for over four seconds. Their method can help improve the accuracy of quantum sensors, where both the number of trapped atoms and the trapping time are crucial. Such quantum systems are used to study dark matter, refine navigation systems, and aid in mineral exploration. The study findings have been published in the Journal of Experimental and Theoretical Physics Letters.